Data science has become a critical tool for businesses to unlock value from their data. This article explores real-world case studies from industries like retail, healthcare, and finance, showcasing how companies such as Walmart and JPMorgan Chase use data science to drive growth, optimize operations, and enhance customer experiences.
Table of Contents
- Retail Industry: Walmart’s Demand Forecasting with Data Science
- Healthcare: Personalized Medicine at Mount Sinai Hospital
- Financial Services: Credit Risk Management at JPMorgan Chase
- Transportation: Optimizing Delivery Routes at UPS
- Marketing and Customer Engagement: Netflix’s Personalized Recommendations
- Conclusion
Data science has emerged as one of the most powerful tools for businesses seeking to harness the value of their data. It is not just a trend but a necessity for organizations aiming to stay competitive in the digital age. By turning raw data into actionable insights, companies can optimize operations, make informed decisions, and drive sustainable growth.
This write-up explores real-world case studies that showcase how businesses have successfully applied data science to solve key challenges, improve performance, and achieve measurable growth. These examples span industries such as retail, finance, healthcare, and transportation, highlighting the versatility and transformative power of data science.
1. Retail Industry: Walmart’s Demand Forecasting with Data Science
Retail companies thrive on predicting demand accurately to maintain optimal inventory levels and prevent stockouts or overstock. Walmart, the world’s largest retailer, has leveraged data science to optimize demand forecasting and inventory management.
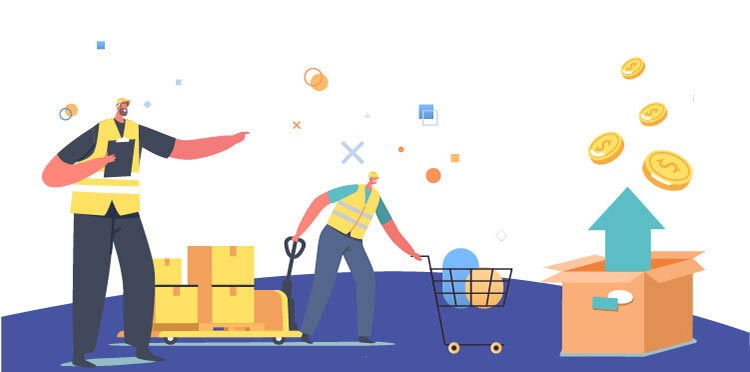
The Challenge
Walmart operates thousands of stores worldwide, offering millions of products. The challenge lay in predicting demand for each product in each store, considering factors such as local holidays, weather conditions, and promotional events. Manual forecasting methods couldn’t keep up with the complexity and volume of data.
The Solution
Walmart developed a machine learning-based demand forecasting model using data science techniques like time-series analysis, regression models, and neural networks. The model takes into account historical sales data, economic factors, weather data, and social media trends. By using advanced algorithms, Walmart can now forecast demand at a granular level, improving its inventory management processes.
Results
- Reduction in Stockouts: Walmart reported a significant reduction in stockouts (when products are unavailable), leading to increased sales and customer satisfaction.
- Improved Inventory Turnover: The more accurate demand forecasts led to optimized inventory levels, reducing overstock and associated costs.
- Revenue Growth: By preventing lost sales due to stockouts and reducing excess inventory costs, Walmart achieved measurable growth in revenue and profit margins.
Key Insight
Data science-driven forecasting not only optimizes operations but also has a direct impact on revenue by enhancing customer experience and reducing operational waste.
2. Healthcare: Personalized Medicine at Mount Sinai Hospital
The healthcare industry is one of the most data-intensive sectors, with patient records, treatment histories, and diagnostic information generating massive amounts of data daily. One of the most groundbreaking applications of data science in healthcare is the development of personalized medicine, where treatments are tailored to individual patients.
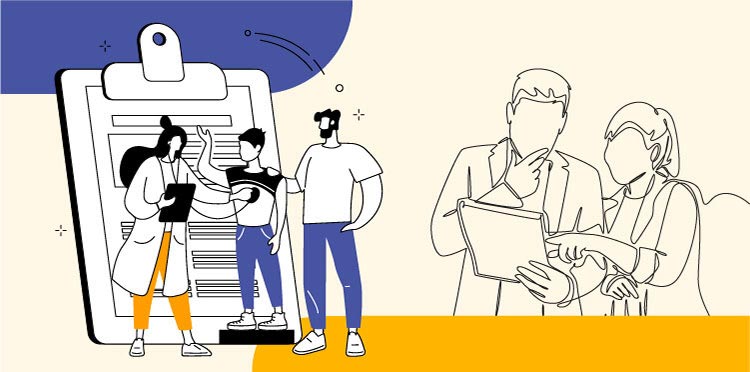
The Challenge
Mount Sinai Hospital in New York aimed to improve patient outcomes by identifying personalized treatment plans for individuals, especially for complex diseases such as cancer and cardiovascular conditions. The challenge lay in making sense of the vast amounts of patient data and genomic information to create tailored treatment strategies.
The Solution
Mount Sinai leveraged machine learning algorithms and predictive analytics to analyze vast datasets, including patients’ medical histories, genomic data, and clinical trial results. By applying data science to patient data, they were able to develop predictive models that identified the most effective treatment options for each patient.
In particular, the AI-driven predictive models identified patterns in genetic data that indicated which treatments were likely to work best for patients with specific genetic profiles.
Results
- Improved Treatment Outcomes: Patients receiving personalized treatment plans based on data analysis had higher success rates in treatment.
- Reduced Trial-and-Error: Doctors no longer needed to rely on trial-and-error for treatments, as data-driven insights provided better guidance on effective therapies.
- Cost Reduction: Personalized medicine reduced the cost of care by minimizing unnecessary treatments and hospital readmissions.
Key Insight
Personalized medicine, enabled by data science, represents a paradigm shift in healthcare, where treatments are tailored to the individual rather than a one-size-fits-all approach, leading to better patient outcomes and cost efficiency.
3. Financial Services: Credit Risk Management at JPMorgan Chase
In the financial services sector, managing credit risk is critical to maintaining profitability while minimizing losses. Traditional methods of assessing creditworthiness were limited by reliance on historical data and manual assessments. JPMorgan Chase, one of the largest banks in the world, turned to data science to improve its credit risk management processes.
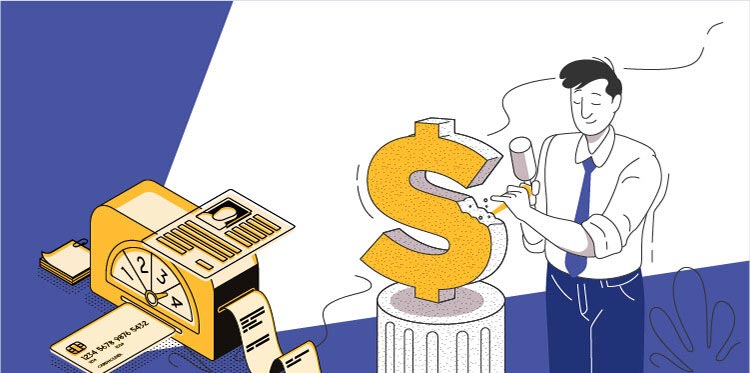
The Challenge
JPMorgan Chase faced the challenge of accurately assessing credit risk for millions of loan applications while reducing the time it took to approve or reject loans. The traditional methods were time-consuming, lacked precision, and didn’t account for the vast number of variables that could predict risk.
The Solution
The bank deployed machine learning models to improve the accuracy of credit scoring and risk assessment. Using data science techniques such as logistic regression, decision trees, and deep learning, JPMorgan Chase’s models analyzed a wide range of data points, including:
- Financial history: Transaction history, credit card usage, and loan repayment behaviors.
- Demographics: Age, income level, and employment status.
- Social media and Digital Footprints: Non-traditional data sources were used to capture insights that traditional credit scores might miss.
Results:
- Faster Decision-Making: Loan approvals were accelerated, with automation significantly reducing the time it took to make decisions on creditworthiness.
- Reduced Default Rates: The accuracy of the risk models helped reduce default rates by identifying high-risk applicants more effectively.
- Increased Loan Approvals: More customers were approved for loans as the models could accurately identify low-risk customers who may have been overlooked by traditional methods.
Key Insight:
Data science in financial services not only improves credit risk management but also enables better customer service by speeding up processes and offering more personalized financial products.
4. Transportation: Optimizing Delivery Routes at UPS
In the logistics and transportation industry, optimizing delivery routes is essential for cutting costs and improving efficiency. UPS (United Parcel Service) is a leader in applying data science to improve delivery operations, and their ORION system (On-Road Integrated Optimization and Navigation) is a prime example of data science in action.
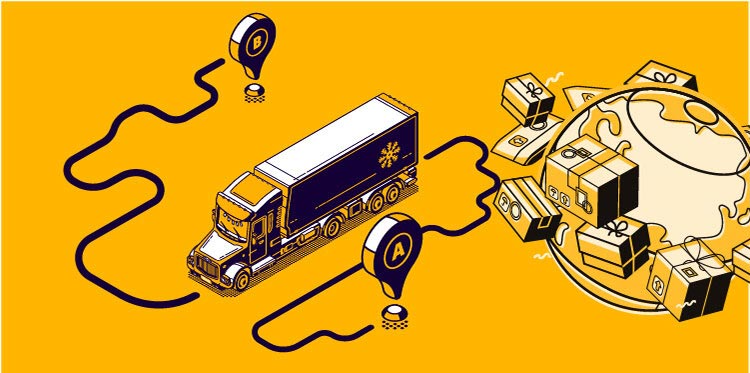
The Challenge
UPS drivers make millions of deliveries every day, and the challenge was to find the most efficient routes that would reduce fuel consumption, delivery times, and operational costs. With millions of potential delivery routes and variables such as traffic, weather, and package type, manual route optimization was inefficient.
The Solution
UPS implemented ORION, a data-driven system powered by algorithms and advanced analytics to calculate the most efficient routes for drivers. ORION considers various data sources, including:
- Real-time traffic data: To avoid congestion and accidents.
- Delivery windows: Ensuring that high-priority packages are delivered on time.
- Vehicle capacities: Optimizing the loading and unloading process.
Results:
- Reduction in Fuel Consumption: By optimizing delivery routes, UPS saved over 10 million gallons of fuel annually.
- Cost Savings: UPS reported savings of over $400 million per year due to reduced fuel usage and more efficient delivery operations.
- Improved Customer Satisfaction: Faster and more reliable deliveries led to improved customer satisfaction.
Key Insight:
By using data science to optimize logistics and transportation, companies can achieve significant cost savings and improve service quality, creating a competitive advantage in an industry where margins are thin.
5. Marketing and Customer Engagement: Netflix’s Personalized Recommendations
In the world of streaming entertainment, Netflix has set the gold standard for customer engagement by leveraging data science to offer personalized content recommendations. Netflix’s recommendation engine is one of the most well-known applications of data science in the media industry.
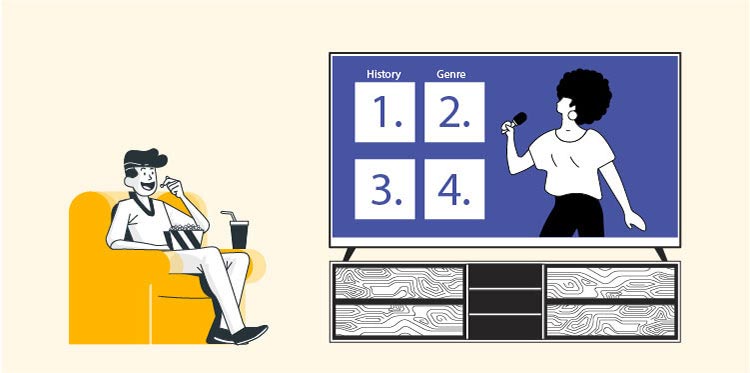
The Challenge
With a vast library of content and millions of subscribers, Netflix needed a way to keep users engaged and reduce churn by recommending relevant movies and TV shows. Manual curation was not feasible, and there was a need for a personalized, data-driven approach.
The Solution
Netflix built a collaborative filtering recommendation engine that uses data science algorithms to suggest content based on users’ viewing history, preferences, and behavior. The engine analyzes data points such as:
- Viewing history: What users watched and how they rated the content.
- User interactions: Browsing history, pauses, rewinds, and clicks.
- Content characteristics: Genres, actors, directors, and themes.
By leveraging machine learning and natural language processing (NLP), the recommendation engine continuously learns and adapts to individual user preferences.
Results:
- Increased User Engagement: Personalized recommendations have been shown to increase viewing time and keep users engaged with the platform.
- Reduction in Churn: The personalized experience has helped Netflix retain subscribers by consistently offering relevant content.
- Revenue Growth: Increased user engagement and retention contributed to significant revenue growth as Netflix expanded its global subscriber base.
Key Insight:
Personalized recommendations, powered by data science, enhance the customer experience by offering tailored content that keeps users engaged, leading to higher retention and business growth.
Conclusion
The case studies outlined above demonstrate how data science is transforming industries by providing businesses with actionable insights that drive growth. From retail giants optimizing their supply chains to healthcare providers personalizing treatment plans, the ability to harness data effectively is a key differentiator for companies in today’s competitive landscape.
As the use of data science continues to evolve, businesses that embrace these technologies will be better positioned to innovate, adapt, and grow in the ever-changing market environment. Data science, when applied strategically, has the potential to unlock new opportunities, improve customer experiences, and generate substantial business value. In a world where data is a strategic asset, organizations that successfully leverage data science will lead the charge in shaping the future of their industries.
← Back to Blog