Discover how demand forecasting analytics optimizes manufacturing supply chains by enhancing production planning, inventory management, and customer satisfaction while reducing costs and driving strategic decisions.
Table of Contents
- What is Demand Forecasting Analytics?
- Why Demand Forecasting Matters in Manufacturing
- 3 Methods of Demand Forecasting in Manufacturing
- 5 Ways to Implement Demand Forecasting Analytics in Manufacturing
- Benefits of Demand Forecasting Analytics in Manufacturing
- Real-World Applications and Case Studies
- Future Trends in Demand Forecasting Analytics
- Conclusion
In the realm of manufacturing, optimizing the supply chain is a critical endeavour. The ability to predict demand accurately is paramount for efficient production planning, inventory management, and customer satisfaction. This is where demand forecasting analytics comes into play, revolutionizing how manufacturers approach the complexities of the supply chain. In this article, we delve into the significance of demand forecasting, its impact on the manufacturing supply chain, and how it drives efficiency and competitive advantage.
What is Demand Forecasting Analytics?
Demand forecasting analytics involves the use of advanced statistical methods, machine learning algorithms, and data analysis techniques to predict future customer demand. By leveraging historical sales data, market trends, economic indicators, and other relevant factors, demand forecasting provides businesses with actionable insights that guide decision-making processes.
Why Demand Forecasting Matters in Manufacturing
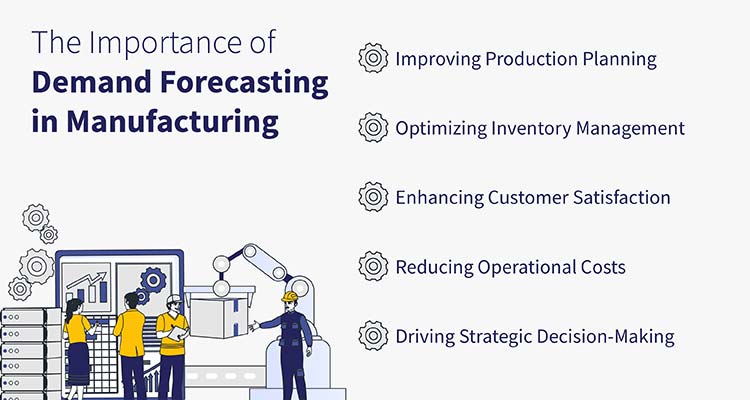
Improving Production Planning
Accurate demand forecasts enable manufacturers to plan production schedules effectively, ensuring that they produce the right quantity of products to meet customer demand without overproduction or stockouts.
Optimizing Inventory Management
By predicting future demand, manufacturers can optimize inventory levels, reducing carrying costs & minimizing the risk of obsolete stock. This leads to better utilization of warehouse space & financial resources.
Enhancing Customer Satisfaction
Meeting customer demand on time and in full is crucial for maintaining customer satisfaction and loyalty. Demand forecasting helps manufacturers anticipate customer needs and respond proactively.
Reducing Operational Costs
Accurate demand forecasting allows manufacturers to align their supply chain activities, reducing unnecessary costs related to excess inventory, expedited shipping, and production downtime.
Driving Strategic Decision-Making
Demand forecasting analytics provides insights that support strategic decision-making, such as market expansion, product development, and pricing strategies.
3 Methods of Demand Forecasting in Manufacturing
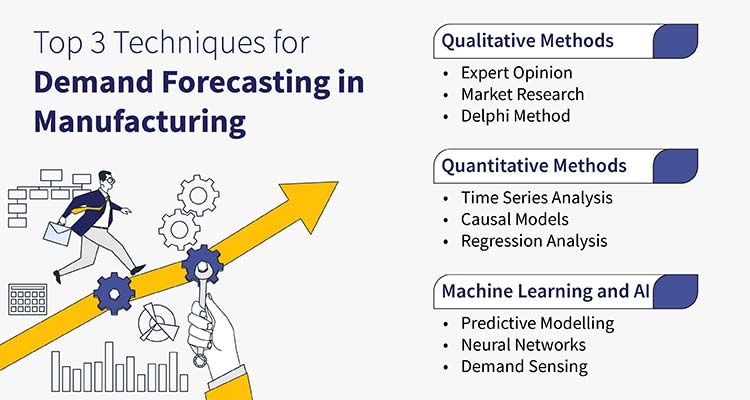
1. Qualitative Methods
- Expert Opinion: Gathering insights from industry experts, sales teams, and key stakeholders to forecast demand based on their knowledge and experience.
- Market Research: Conducting surveys, focus groups, and customer interviews to understand future demand trends and preferences.
- Delphi Method: Using a panel of experts to reach a consensus on future demand through iterative rounds of questionnaires and feedback.
2. Quantitative Methods
- Time Series Analysis: Using historical data to identify patterns and trends over time, which can be extrapolated to forecast future demand.
- Causal Models: Identifying relationships between demand and external factors such as economic indicators, marketing activities, and seasonal effects.
- Regression Analysis: Utilizing statistical techniques to model the relationship between demand and independent variables, enabling precise demand predictions.
3. Machine Learning and AI
- Predictive Modelling: Leveraging machine learning algorithms to analyze vast datasets and generate accurate demand forecasts based on historical patterns and real-time data.
- Neural Networks: Using deep learning techniques to capture complex relationships and interactions within data, providing robust demand forecasts.
- Demand Sensing: Employing real-time data from various sources such as social media, market trends, and point-of-sale systems to capture short-term demand signals and improve forecast accuracy.
5 Ways to Implement Demand Forecasting Analytics in Manufacturing
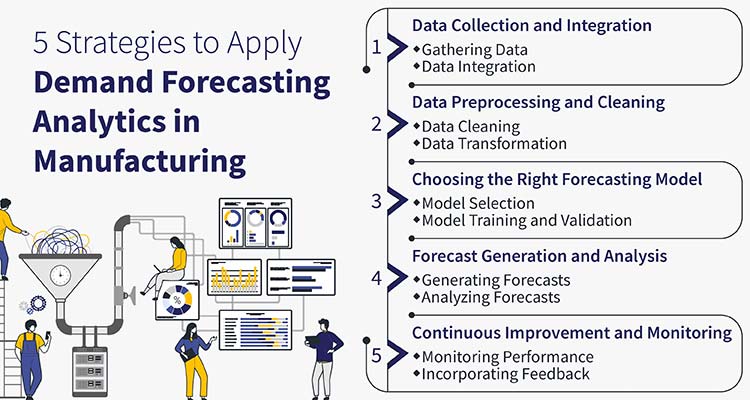
1. Data Collection and Integration
- Gathering Data: Collecting relevant data from various sources, including historical sales data, customer orders, market trends, and external factors.
- Data Integration: Integrating data from different systems and departments to create a unified dataset for analysis.
2. Data Preprocessing and Cleaning
- Data Cleaning: Removing inaccuracies, outliers, & inconsistencies from the dataset to ensure high-quality data.
- Data Transformation: Normalizing and transforming data to make it suitable for analysis and modeling.
3. Choosing the Right Forecasting Model
- Model Selection: Selecting the appropriate forecasting model based on the nature of the data, forecasting horizon, and business requirements.
- Model Training and Validation: Training the selected model using historical data & validating accuracy through back-testing & evaluation.
4. Forecast Generation and Analysis
- Generating Forecasts: Running the forecasting model to generate demand forecasts for different time periods and scenarios.
- Analyzing Forecasts: Analyzing the generated forecasts to identify patterns, trends, and potential anomalies.
5. Continuous Improvement and Monitoring
- Monitoring Performance: Continuously monitoring the performance of the forecasting model and making adjustments as needed to improve accuracy.
- Incorporating Feedback: Gathering feedback from stakeholders and incorporating it into the forecasting process to enhance its reliability and relevance.
Benefits of Demand Forecasting Analytics in Manufacturing
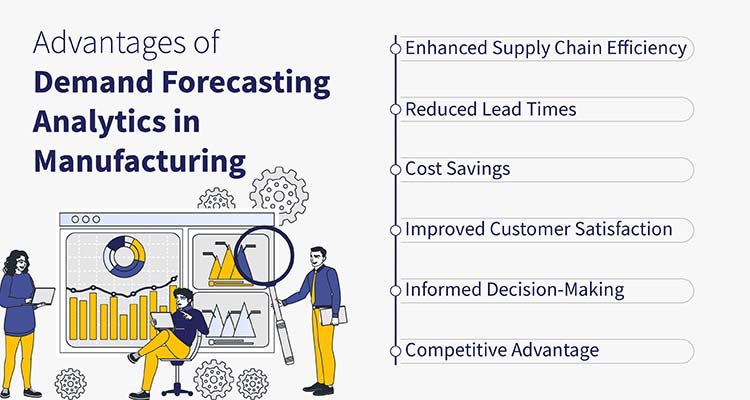
- Enhanced Supply Chain Efficiency: Demand forecasting analytics enables manufacturers to synchronize their supply chain activities, ensuring that raw materials, production schedules, and distribution plans are aligned with actual demand.
- Reduced Lead Times: By accurately predicting demand, manufacturers can streamline their operations, reduce lead times, and improve responsiveness to customer orders.
- Cost Savings: Optimizing inventory levels and production schedules leads to significant cost savings by minimizing excess inventory, reducing storage costs, and avoiding production inefficiencies.
- Improved Customer Satisfaction: Meeting customer demand consistently and efficiently enhances customer satisfaction, leading to increased loyalty and repeat business.
- Informed Decision-Making: Demand forecasting analytics provides valuable insights that support strategic decision-making, enabling manufacturers to adapt to market changes and seize new opportunities.
- Competitive Advantage: Leveraging advanced demand forecasting techniques gives manufacturers a competitive edge by enabling them to anticipate market trends, respond proactively to customer needs, and stay ahead of competitors.
Real-World Applications and Case Studies
1. Case Study: Automotive Industry
Challenge: An automotive manufacturer faced challenges in predicting demand for different vehicle models, leading to production inefficiencies and stockouts.
Solution: Implementing a demand forecasting analytics solution that integrated historical sales data, market trends, and economic indicators to generate accurate demand forecasts.
Outcome: The manufacturer improved production planning, reduced lead times, and increased customer satisfaction by consistently meeting demand for different vehicle models.
2. Case Study: Consumer Electronics
Challenge: A consumer electronics company struggled with inventory management due to fluctuating demand and rapid product obsolescence.
Utilizing machine learning algorithms to analyze sales data, market trends, and customer preferences, enabling precise demand predictions for different product lines.
Outcome: The company optimized inventory levels, reduced carrying costs, and minimized the risk of obsolete stock, leading to higher profitability and customer satisfaction.
3. Case Study: Retail Industry
Challenge: A retail chain experienced difficulties in forecasting demand for seasonal products, resulting in stockouts and lost sales.
Solution: Implementing a demand sensing solution that leveraged real-time data from social media, market trends, and point-of-sale systems to capture short-term demand signals.
Outcome: The retail chain improved forecast accuracy, reduced stockouts, and increased sales by aligning inventory levels with actual customer demand.
Future Trends in Demand Forecasting Analytics
Integration of Big Data and IoT
The integration of big data and Internet of Things (IoT) technologies will provide manufacturers with real-time insights into demand patterns, enabling more accurate and dynamic forecasting.
Advanced Machine Learning Techniques
The use of advanced machine learning techniques, such as reinforcement learning and ensemble methods, will further enhance the accuracy and reliability of demand forecasts.
Collaborative Forecasting
Collaborative forecasting involves integrating data and insights from various stakeholders, including suppliers, distributors, and customers, to create more comprehensive and accurate demand forecasts.
AI-Driven Automation
AI-driven automation will streamline the demand forecasting process, enabling manufacturers to generate and analyse forecasts more efficiently and make data-driven decisions in real-time.
Predictive and Prescriptive Analytics
The combination of predictive and prescriptive analytics will enable manufacturers to not only forecast demand but also recommend optimal actions to meet that demand, further optimizing the supply chain.
Conclusion
Demand forecasting analytics is a powerful tool that can transform the manufacturing supply chain. By leveraging advanced analytics techniques and technologies, manufacturers can predict customer demand with greater accuracy, optimize production and inventory management, and enhance customer satisfaction.
The benefits of demand forecasting analytics extend beyond operational efficiency, driving strategic decision-making, cost savings, and competitive advantage. As the manufacturing landscape continues to evolve, embracing demand forecasting analytics will be essential for staying ahead of the competition and achieving long-term success.
← Back to Blog